The “AI is unethical” myth
I am never surprised by online hype. The hype bicycle which many Data Privacy and other professionals are riding at the moment is that AI creates machines that have human intelligence, is very dangerous to humanity, and is unethical.
So, once you’ve unlocked your phone or computer using your face or your fingerprint (using AI that has been around since 2011) to read this article, let us consider a few things.
A few points about AI
- The outcomes generated from Machine Learning (ML) are done so using mathematical equations.
- I know of no mathematical equation that can produce human emotions or interpersonal and intrapersonal skills like those of the over 8 billion people who live in the world.
- ML outcomes are no more ethical than the differing beliefs of the 8 billion humans in the world. Any ethical/unethical outcome is simply driven by the mathematical algorithm developed by the developer and the source of the data.
- These mathematical equations produce a probability that an answer is right – note the word “probability” – AI has never claimed 100% accuracy, just like humans.
- As for the ethical outcomes of AI, we should remember: there are some scientists around the world producing lifesaving drugs, and some others producing drugs and gases that could kill millions. Human beings have always exploited revolutionary inventions for unethical purposes. Unethical to one person can be ethical to some. We are all unique.
How does AI work?
A simple example of AI in practice is if we look at fingerprinting to open your computer or phone.
When first set up, you’re instructed to scan your fingerprint many times. The software algorithm scans the unique fingerprint ridges and peaks and assigns each of them a number. This is replicated hundreds of times until your fingerprint has a number combination – just like a combination padlock. Then when you scan your finger to open your computer, it analyses your print, assigns numerical values to ridges and peaks using the grid method, and matches it with the stored set of numbers that reside in the database that match you, and there. Unlocked.
So in simple terms, ML uses mathematics to deduce a probable outcome.
To learn in more detail about how fingerprint scanners work, you can visit this webpage.
Introducing Bayesian theory
One such probability theory that data privacy practitioners need to be aware of is Bayesian theory. This will allow you to understand that AI outcomes are just that – decisions made that are based on a high mathematical probability.
Just like weather forecasts.
How many times have you looked at an AI-generated weather forecast that predicted a dry day, then left the house without a coat and it started to rain – which was never in the forecast? That’s because the forecast is based upon probability, and thus won’t always be spot on.
It looks and sounds complicated but let me explain it in simple terms.
Bayesian theory is a way to make smart guesses or predictions. Imagine you’re trying to figure out if it will rain tomorrow. You start with a guess based on what you already know. Then, as you gather more information, you adjust your guess to be more accurate.
Bayesian theory in AI
AI uses Bayesian theory to make decisions by combining new information with what it has learned from past experiences. Think of it like teaching a computer to learn from examples. AI starts with a rough idea and keeps getting better at guessing as it learns from more examples.
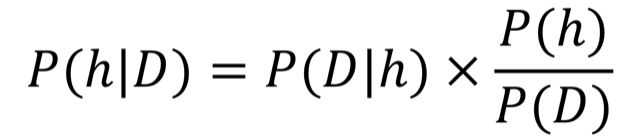
What can factor into AI making mistakes?
AI can’t always be right with Bayesian or other probability theories. And here’s why:
Limited Knowledge
AI’s guesses are only as good as the information it has. If it hasn’t seen something before, there’s a chance it might not guess very well.
Imperfect Data
AI learns from data. However, if the data is wrong or biased, its guesses can be off. Imagine if it learned only from sunny days. Its guesses on rainy days might not be accurate as a result.
Assumptions Matter
AI makes assumptions to guess. If these assumptions are wrong, their predictions can be, too.
Changes and Surprises
AI doesn’t always know when things change. So, if something unexpected happens, its guess might be wrong.
Complexity Confusion
Some things are really tricky to predict, like stock markets. AI might get confused with these complex situations.
Rare Events
If something happens super rarely, AI might not guess it right because it hasn’t seen it much before.
Human Errors
If people make mistakes while teaching the AI, its guesses can also be wrong.
Ethics and Feelings
AI doesn’t understand ethics or human feelings, which can affect predictions in situations where these matter.
No Understanding of Context
AI lacks genuine understanding and context. It might make predictions that sound accurate but don’t make sense in the real world.
Adversarial Examples
AI can be fooled by intentionally crafted inputs that humans wouldn’t find confusing. This shows that AI’s understanding is fundamentally different from human understanding.
Computational Limits
AI processes a vast amount of data and computations, but there are limits to its capabilities due to computing power and time constraints.
Artificial Intelligence isn’t perfect
Remember, AI is like a smart friend. It tries its best to guess, and can often make solid predictions based on its experiences, but it’s not perfect. Because of the reasons above, it can’t be 100% accurate all of the time.
It can, however, just like humans, try its best.
By Nigel Gooding LLM, FBCS